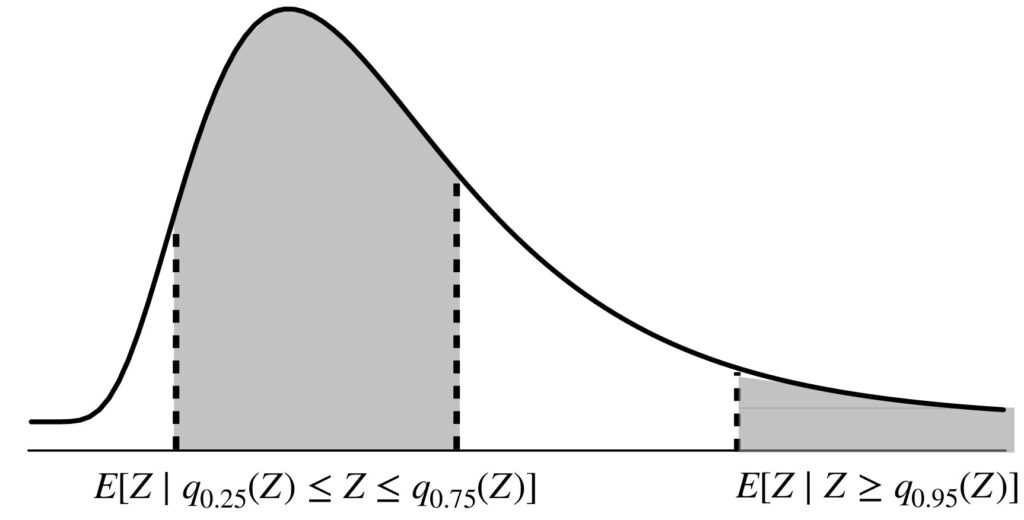
Empowering Climate Science Through Effective Tail Learniing
Project Aims:
- Establish the statistical foundation for integrated-quantile regression and expected shortfall.
- Develop new tail regression methods to estimate climate extremes.
- Explore general tail measures of joint climate extremes.
- Develop and maintain comprehensive platform of climate risk predictions.
Senior Personnel:
- Bo Li, Department of Statistics, University of Illinois at Urbana-Champaign
- Spatiotemporal and environmental statistics
- Huixia Judy Wang, Department of Statistics, George Washington University
- quantile regression, extreme value theory, semiparametric regression, spatial analysis, highdimensional inference
- Kean Ming Tan, Department of Statistics, University of Michigan
- Multivariate statistical methods development
- Ryan Sriver, Department of Atmospheric Sciences, University of illinois at Urbana-Champaign
- Climate and weather extremes
- Wenxin Zhou, Department of Information and Decision Sciences, University of Illinois at Chicago
- High-dimensional data analysis
Recent Highlights:
Undergraduate researcher Ziyi Zhou is developing new expected shortfall techniques to analyze how extreme temperature and precipitation are changing with climate. The figure below shows seasonal trends in the >90% tail of daily temperature distributions since 1940, highlighting increases in summer extremes within much of the western US.